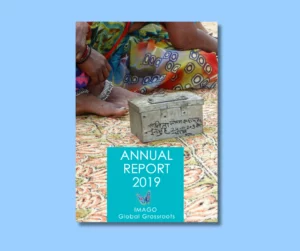
Annual Reports
2019 Annual Report
Mar 31st 2020
To read the 2019 annual report, click here.
Read MoreIn August 2020, schools all around the world were debating a complex issue, whether or not to go back to in-person classes. The answer was not evident then and is still not evident now. The most common tension was between the relevant national or local public health authority’s advice, parents’ demands, and teachers’ concerns about getting infected. Any decision to go back to in-person classes or to implement a hybrid model would largely depend on the evolution of COVID-19 cases.
With so many unknown factors, it was nearly impossible to make the right decision that could be sustained for the entire school year. Under such circumstances, how could we evaluate the proposed policy to make sure it has the desired impact on learning? A standard impact evaluation would require extensive data collection, baseline information, and a follow-up survey that would probably take at least one year to show preliminary causal results. A standard evaluation could give us the precise impact of going back to in-person classes on students’ learning, but it would not show us what implementation processes worked best, or what contextual factors made the policy more effective. For example, a standard evaluation would not show us if political stability at the school district was the key to success or if having access to free rapid testing was the main enabling factor. Also, a standard evaluation would not allow us to improve our understanding of how the intervention interacted with the ecosystem. For instance, it would not show us if particular stakeholders, such as parents or teachers, needed special consideration in order to be onboarded with the decision of going back to in-person classes. Moreover, a standard evaluation would not allow us to test for other potential solutions that could have been less costly or less risky than in-person classes.
COVID is just one illustration of the nature of problems faced by policymakers on a day-to-day basis. Most policy decisions are typically sensitive to unexpected human reactions, involve engaging with multiple stakeholders and are largely dependent on the economic and political climate. In this situation, any proposed policy solution would need to be subject to short periodic reviews that little by little would allow us to get closer to the best approach.
⚬ ⚬ ⚬
Adaptive Evaluations are designed to answer some of the most intractable, and often perplexing questions that policymakers tend to face. We call these thorny and complex questions adaptive questions because they require adjusting to unpredictable human behavior, systems, and contexts. An adaptive evaluation is designed to answer the four types of questions described in Figure 1: theory of change, system readiness, optimal design, and questions on impact and effectiveness. Answering these questions in complex setting requires different approaches; we describe each of them below.
Theory-based approaches answer questions pertaining to the theory of change. They help unpack the black box of a program, the often-ambiguous space between the actual intervention and the desired outcomes. Theory-based approaches allow us to consider multiple pathways from the intervention to the outcomes, but also to look into interactions between the pathways, and potential unintended consequences. A typical starting point for such an approach includes the use of Fishbone or Ishikawa diagrams (see Figure 2), followed by a theorization of the potential causal pathways along different channels.
System-based approaches are designed to answer questions on system behavior—the multiple interactions within a system, as well as overall system change—including assessment of system readiness for particular interventions. They help understand the key stakeholders that drive systems, and the enablers and resistors of change. They also help identify reinforcing positive and/or negative feedback loops at play as well as the demands of an intervention on the system. Typical techniques involve system maps and diagnostics.
Iterative approaches answer optimal design and proof of concept questions and are central to the conception of an adaptive evaluation. They are intrinsically adaptive in that they require an evaluator to constantly tweak and refine design in response to feedback. The main features of this approach include rapid prototyping, experimentation, and frequent testing, in close collaboration with implementers. The emphasis here is on learning frequently in short sprints and adapting the intervention to the learning as quickly as possible. A range of techniques can be used in iteration, including A/B testing[i], process tracing[ii] and beneficiary journeys.
Note the idea of iterative adaptation is frequently used in the private sector, and less commonly in the public sector. Specific methods, such as design-thinking and agile, are highly developed in the technology sector, but now are increasingly spreading to other industries. Most technology start-ups use some form of adaptive evaluation on a regular basis. It is another way of learning how to try fast and fail fast. Through this approach, they are able to iterate and navigate the system as they develop their business model, without spending too many resources on the way.
Finally, for questions of impact and effectiveness, the fourth element of adaptive evaluation is the systematic use of mixed-method approaches. This consists of the use of quantitative and qualitative evidence with each informing the design of the other, allowing a more holistic interpretation of the results. This makes the evaluation more robust especially when some aspects are difficult to measure (such as satisfaction or political buy-in) or in situations when results are highly sensitive to the context and conditions.
Image Sources: Theory-Based (top and bottom), Systems-Based, Iterative (top and bottom) , Mixed-Methods
Adaptive evaluations differ from standard evaluations not only in the questions they tackle, but also in its underlying philosophy. Adaptive evaluations do not presuppose a hypothesis or a technique ex-ante but consider multiple hypotheses. They are open-minded with methods, as they realize that different questions require different tools. Perhaps more pertinently, adaptive evaluations transition from a testing environment, typically found in standard impact evaluations, to a learning environment, where the aim is not to verify an outcome but to learn the best way to achieve it.
There is also a major difference in objectives, positioning, and reporting. Standard evaluations focus on precise testing of a specific hypothesis[1] pertaining to an intervention with conditions remaining the same (ceteris paribus), while adaptive evaluations focus on continuous learning by assessing multiple interventions and several potential hypotheses in dynamic environments (mutatis mutandis). An evaluator in a standard evaluation would position themselves as an objective and independent outsider, attempting only to observe and document what the intervention team does, while being careful not to influence them. On the other hand, an adaptive evaluation is part of the internal evaluation unit, embedded within the intervention team and integrated with the process, design, improvement, and scaling of the program. Finally, a standard evaluation report results in academic papers that often take years to be published—with 5 years not uncommon, with an emphasis on internal validity (i.e., the rigor of causal attribution).[iii] On the contrary, an adaptive evaluation, reports learning in more accessible reports/papers published every 6 months, with an emphasis on external validity (i.e., the context).[iv]
We see adaptive evaluation as a complement to standard evaluation tools, rather than a substitute. We need both approaches as long as we are clear what we are using when. An excellent example of adaptive and standard evaluations working in tandem is Pratham, which over the last two decades has used a range of evaluation tools to develop the Teaching at the Right Level program. Pratham was set up with evidence at the center. From the beginning, they invested in organizational capacity for Monitoring and Evaluation, and they implemented a strong unit to standardize and streamline data collection and analysis. They used information to continuously learn from their various tutoring programs and innovative teaching models, conducting frequent pilots that were iterative and focused on learning and optimal design. As the designs matured, they used RCTs to test whether their design worked. They also took advantage of this information to engage various stakeholders in their scaling up process, from government officials to internal staff members.
As one can imagine, an adaptive evaluation comes with its own set of challenges, different from a standard evaluation. An adaptive evaluation demands a high level of trust, communication and coordination between the evaluation and implementation teams. This is not a trivial task, since it involves multiple stakeholders to align their interest and pivot their organizations to learn as opposed to test, and to prototype as opposed to deploy. It requires a unique type of leadership of the implementing organization and evaluation team, which is also adaptive. Adaptive leadership implies a shift of mindset from leaders providing solutions to enabling environments for collaboration, motivation, and mobilization of different talents to enact meaningful change. Another challenge of adaptive evaluations is managing multiple hypotheses while maintaining the capacity to be nimble and adapt to the evidence. Nonetheless, with the appropriate internal capacity, leadership and holding environment, adaptive evaluations answer the core questions that help address difficult policy problems.
⚬ ⚬ ⚬
IMAGO’s adaptive evaluations were developed as a natural complement to the work it has been doing for the past 8 years on scaling innovations with grassroots organizations. Our implementation work typically involves management techniques, some of which are similar in approach and spirit to implementation strategies such as Agile or Problem Driven Iterative Adaptation. Our experience is that scaling up involves the transformation of an organization, which is not a linear process and has unpredictable outcomes. It also involves adaptive leadership with no easy answers. Standard evaluations were not designed for these types of change processes, even though rapid RCTs are a useful tool for some of the stages in the process of scaling up.
At IMAGO, we are working on two large-scale adaptive evaluations. The first is an evaluation of an Enterprise Support System (ESS) for the artisanal, credit, and farmer-producer enterprises owned by members of the Self-Employed Women Association (SEWA) in India. The ESS involves multiple interventions, such as increased access to mentor networks, management support (coaching and leadership), financial support, and knowledge management. The evaluation involves prototyping multiple designs to understand what kind of ESS can help the SEWA enterprises achieve financial sustainability as well as improve the agency of its women members. Since the ESS involves multiple interventions with several complementarities that interact with the behavior and motivations of a large realm of stakeholders and involves iterative exploration of what does and does not work, an adaptive evaluation is a natural fit.
More recently, IMAGO started work on a multi-year Monitoring, Learning, and Evaluation grant to generate evidence on the effectiveness of developing convergence mechanisms between economic and social programs for driving women’s economic empowerment in India. The BMGF grant involves working closely with the state governments in Madhya Pradesh and Bihar, alongside our implementation partner, Transform Rural India, and our data partner, Sambodhi. The interventions focus on linking the activities of federations of self-help groups composed of disadvantaged women with the government’s major rural employment guarantee scheme, as well as helping gender resource centers ensure rural women get access to government services and entitlements. These interventions are designed to scale up through two national programs, NRLM and MGNREGA, once we understand what works and doesn’t, which is better suited to an adaptive evaluation.
⚬ ⚬ ⚬
Policy is convoluted, and inevitably involves not only coordination between multiple stakeholders, but also engaging with systems, and human behavior, which are largely unpredictable. The pandemic has made it abundantly clear how we live in a world that is always evolving. Testing under lab-like settings, while an important part of the learning and evaluation process, is rarely able to capture all the nuance of what needs to be done for a policy to make meaningful transformation in these shifting contexts. Adaptive evaluations are a way of accepting that we will need to adjust on the way as circumstances move. It is a way of remaining humble and open to change when change is needed.
[1] A standard evaluation could in principle have alternative treatment arms for different implementation processes, for example, but these have to be tightly specified before the evaluation starts, held constant through the evaluation and, in any case, limited to a very small number of alternatives.
⚬ ⚬ ⚬
[i] A/B testing is technique of frequent testing which consists of a randomized experimentation process in which two versions of something (i.e., program, intervention, website) are shown to different segments of the population at the same time in order to rapidly determine what worked best in terms of the desired outcomes.
[ii] Process tracing is a qualitative method that uses logic tests to assess the strength of evidence for specified causal relationships, within a single-case design and without a control group.
[iii] Internal validity refers to the degree of confidence that the causal relationship being tested is trustworthy and not influenced by other factors or variables.
[iv] External validity refers to the extent to which results from a study can be applied (generalized) to other situations, groups, or events.